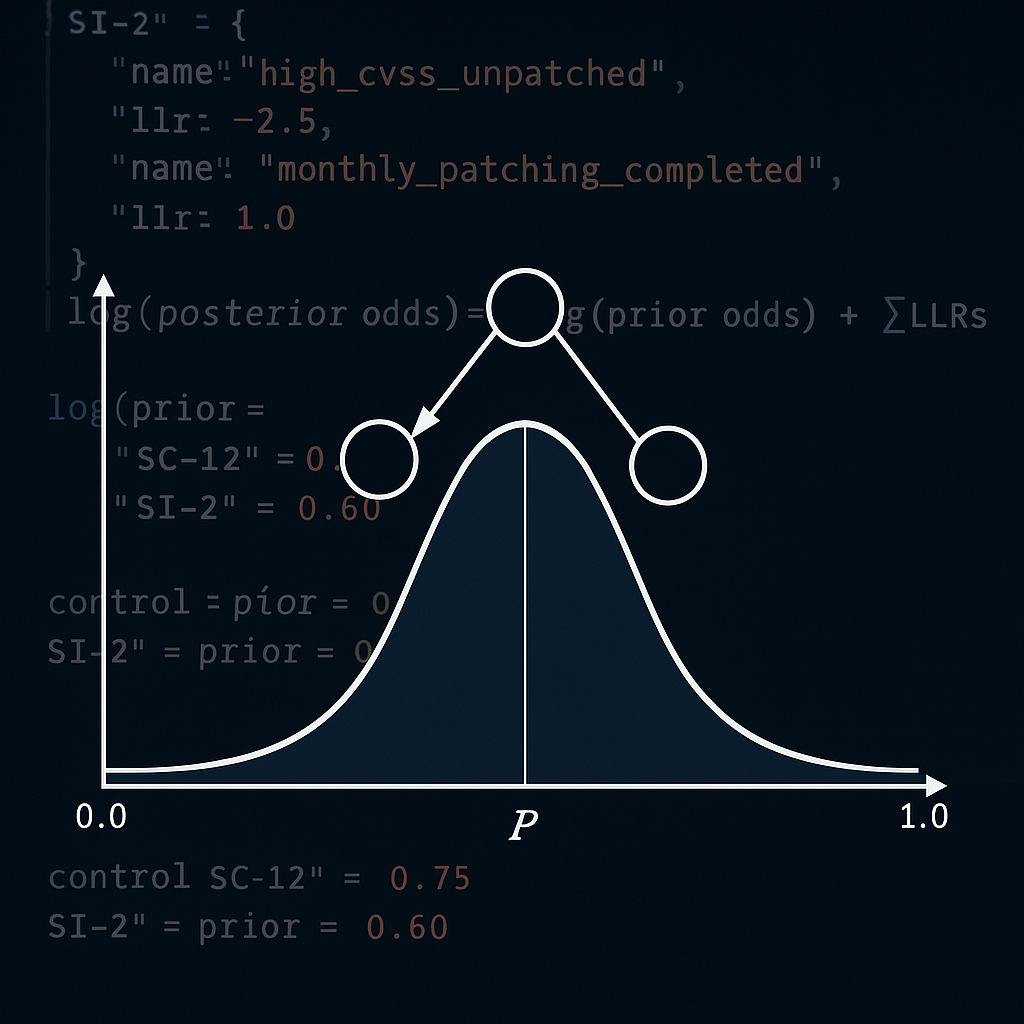
Part 2: Toward Continuous Compliance Quantifying Risk with Bayes and Capturing Evidence in a Security Ledger
Part 2: Toward Continuous Compliance Quantifying Risk with Bayes and Capturing Evidence in a Security Ledger
By Chris Johnson, CTO of Knox Systems
In Part 1, we introduced the Security Ledger—a real-time, tamper-proof system that reframes FedRAMP compliance as a probabilistic, continuously updated measure, not a static report. Now, in Part 2, we go under the hood.
We'll show how Bayesian inference, log-likelihood ratios (LLRs), and ledger-based transparency work together to produce a living risk engine—one that is inspectable, auditable, and mathematically defensible.
And yes, we brought code and real data.
From Binary to Bayesian: Probabilistic Assurance of Control Effectiveness
FedRAMP controls aren’t simply "on" or "off." Their effectiveness shifts with context, evidence, and time. So we treat each control as a probabilistic hypothesis:
P(Control is Effective | Evidence)
This lets us reason continuously over real-world telemetry: IAM logs, patch scans, drift reports, vulnerability findings, and more. The system updates confidence scores in real time—no waiting for annual audits.
Step 1: Assigning Prior Probabilities
Every control begins with a prior belief—a starting point for how likely it is to be effective. These priors are informed by:
- Control category (e.g. access control vs. incident response)
- Historical failure rates
- Threat modeling and exploit severity
- Complexity and likelihood of drift
Example:
{
"AC-2": { "prior": 0.90 },
"SC-12": { "prior": 0.75 },
"SI-2": { "prior": 0.60 }
}
These priors are tunable and evolve with new deployments and observed outcomes.
Step 2: Defining Evidence and LLRs
We define discrete evidence events—findings that either increase or decrease confidence in a control. Each is assigned a log-likelihood ratio (LLR):
log(posterior odds) = log(prior odds) + Σ LLRs
This additive update makes real-time scoring efficient and interpretable.
Example for SI-2 (Flaw Remediation):
"SI-2": {
"evidence": [
{ "name": "high_cvss_unpatched", "llr": -2.5 },
{ "name": "monthly_patching_completed", "llr": 1.0 },
{ "name": "vuln_scanner_stale", "llr": -1.0 }
]
}
LLRs are computed based on empirical data and mapped to actual telemetry triggers.
Real-World Example: AC-2 (Account Management)
From our working model:
- Risk Scenario: A former employee's account is still active and exploited
- P(A): 0.3 (probability of compromise if ineffective)
- Evidence LLRs:
- Account review overdue: -1.2
- No MFA for privileged accounts: -1.5
- Active Directory logs confirm removal: +1.0
- Account review overdue: -1.2
This model is applied to all 323 FedRAMP Moderate controls using structured data and open analysis:
🔗 GitHub Repo: Knox-Gov/nist_bayes_risk_auto
Prioritizing What Matters: The High-Risk Controls
Using this model, we ranked all FedRAMP Moderate controls by severity and potential impact.
The Top 11 High-Risk Controls stood out due to:
- High exploitation risk
- Poor observability without targeted telemetry
- Broad system impact if compromised

These controls form the foundation of our telemetry blueprint—what every system should continuously monitor and score.
Step 3: Continuous Confidence Calculation
Every time Prometheus scrapes a new metric:
- Convert prior to log-odds
- Add up matching LLRs
- Convert back to a probability using the logistic function:
P = 1 / (1 + e^(-log odds))
This produces a dynamic confidence score for each control, updated in real time as evidence changes.
Step 4: Writing to the Security Ledger (Amazon Aurora PostresSQL)
Every update—control ID, evidence, LLRs, and confidence score—is appended as a new, immutable revision to Amazon Aurora PostresSQL, our Security Ledger backend.
Each record includes:
- Control ID
- Timestamps
- Prior and posterior probabilities
- Evidence names + timestamps
- LLR sum
- Operator ID (if manually overridden)
This creates a cryptographically verifiable audit trail. Auditors and agencies can trace any score, see what changed, and confirm whether evidence was valid and in-scope.
Why This Must Be Open
If machines are going to tell us when a control is “healthy,” then the logic behind it must be transparent.
That’s why we’re open-sourcing:
- The LLR control dictionary
- Control-to-evidence mappings
- Assumptions and source data
Just like LLMs disclose model weights and benchmarks, compliance logic must be explainable, auditable, and improvable by the community.
Compliance is too important to be a black box.
Recap: What We’ve Built
- Bayesian engine for dynamic scoring
- Prior and evidence probabilities for every FedRAMP Moderate control
- Identification of top 11 high-risk controls
- Immutable compliance ledger in Amazon Aurora PostresSQL
- Prometheus telemetry mapping in progress
- GitHub: Open LLR control spec
Coming in Part 3:
We’ll go deeper into instrumentation—mapping every FedRAMP Moderate control to Prometheus-compatible metrics and redefining the role of the 3PAO as a real-time verifier of system integrity.
The future of trust is continuous, explainable, and open. Let’s build it together.